The constantly changing nature of the Landscapes we all operate in has significant financial implications for our organisations — and some of these effects are surprisingly counter-intuitive. For example, while every new technological advance is accompanied by warnings about humans being replaced in the workplace, what often happens instead is that new, higher-order systems built on top of those advances demand more people, not fewer, in order to stay competitive.
This well-known pattern — often described as ‘creative destruction’, where old practices are replaced by new ones that require even greater levels of investment and talent — is the focus of this chapter, as we explore six rules of the game and their financial implications.
This well-known pattern — often described as ‘creative destruction’, where old practices are replaced by new ones that require even greater levels of investment and talent — is the focus of this chapter, as we explore six rules of the game and their financial implications.
Fig.114: The Impact of Economic Rules of the Game on Financials
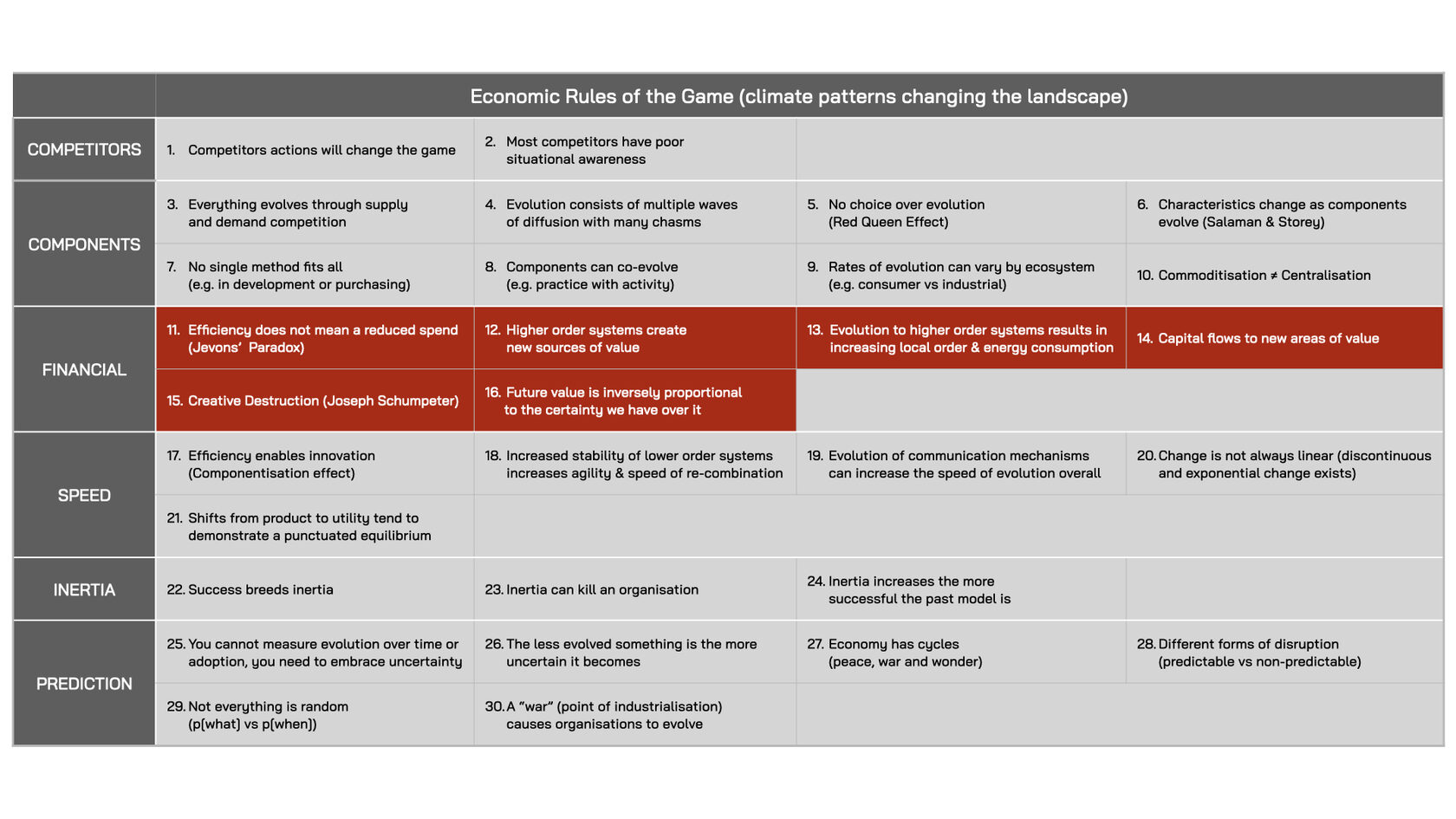
Rule #11. Efficiency Does Not Mean A Reduced Spend (Jevons’ Paradox)
In 1865, the economist William Stanley Jevons published ‘The Coal Question’, in which he explained how Britain’s prosperity during the Industrial Revolution had been fuelled by cheap, high-quality Welsh coal. However, increased efficiency in coal use — most notably from James Watt’s steam engine — did not reduce coal consumption; it increased it. What was once transported slowly and cheaply via canals and horse-drawn transport could now be moved faster and more efficiently by rail.
Jevons warned that the rising demand from increasingly coal-powered industries would deplete Britain’s critical energy resource more rapidly, placing it at a disadvantage compared to rivals with larger coal reserves, such as the United States. This phenomenon — where improvements in resource efficiency lead to higher overall demand for that resource — is known as ‘Jevons’ Paradox’.
The more efficient a resource becomes, the greater the demand for it, as more industries start to depend on it. For example, as cars became more fuel efficient, people drove further because it was more economically viable. This led to changes in practices across other industries (see rule #8), such as the construction of large shopping and entertainment centres outside towns that previously would have been inaccessible. These developments, in turn, encouraged people to drive even more, further increasing energy consumption.
Jevons’ paradox remains relevant today. As the cost per kilometre for electric vehicles declines, people are driving greater distances, thereby ‘offsetting any conservation efforts from improved fuel efficiency1’. The same paradox — where greater efficiency of a component leads to increased use — applies to energy-efficient LED lighting as well. Since LEDs are cheaper to use, homes and offices install more of them, ultimately increasing the consumption of the very energy resources they were intended to conserve.
As components industrialise and become more efficient, demand for them can increase dramatically. This was evident with cloud computing, launched by Amazon in 2006, which provided reliable, cost-efficient computing power that helped trigger the mobile revolution and drive new practices, such as e-commerce, that reshaped traditional industries like retail. However, this growing reliance on widely available, increasingly affordable computing power has significantly increase demand for both electricity (to power data centres) and other natural resources (to build the infrastructure). With the rise of artificial intelligence (AI), this demand is set to increase even further, as more industries integrate these AI-driven technologies into their value chains.
Jevons’ paradox therefore explains how the more efficient a component becomes, the more we use it to enable previously economically unfeasible activities. And, just as coal depletion accelerated in 19th century Britain, (ultimately challenging its role as a global powerhouse), so too will the depletion of the underlying resources modern industries rely on create challenges in the future.
Rule #12. Higher Order Systems Create New Sources Of Value
The availability of reliable, efficient sub-components (e.g. electricity and cloud computing) enables others to experiment with building new, higher-order systems on top of them, which create new sources of value. Electricity enabled the emergence of computers, which eventually led to cloud computing. Today, cloud computing enables the AI revolution by allowing access to powerful models without requiring users to build their own infrastructure first.
New, experimental components in the uncharted space (left of the map) have little certainty about them — neither how to make them reliably nor what they might be used for. Therefore, production costs are high as providers must invest in research and development to figure out how to make these new systems useful and valuable. However, if they find demand, they evolve (rule #3) through the long transitional space (middle of the map). Here, production costs fall as greater certainty emerges around their usefulness and production. This success attracts competitors, who make continuous improvements that help expand the applicable market for the system (rule #4), further stimulating demand and increasing potential profits.
Every successful higher-order system that survives eventually industrialises, moving to the right of the map. At this stage, there’s widespread certainty about what the system (or component) is for and how to produce it. This makes it increasingly difficult for suppliers to differentiate their versions from others, and users become increasingly unwilling to pay more for indistinguishable alternatives. This triggers a race to the bottom on price. What was once a unique and highly-valuable system — such as the first commercially available personal computer, the MITS Altair 8800, for which early adopters paid the equivalent of $3,500 in 1975 — eventually becomes available at a fraction of the price. Value now flows to those best able to exploit economies of scale and provide the volume of components needed at a price users are willing to pay.
However, once these components industrialise, they enable others to build new, higher-order systems on top of them. Reliable and affordable cloud computing enabled everything from navigation (digital maps) to communication (social media) and entertainment (streaming). These higher-order systems have become new sources of value. Today, we don’t compete by building a better cloud computing service — any more than we would try to compete by providing a better electricity network — as there’s virtually no way to differentiate such an offering without incurring prohibitively high costs. Instead, competition lies in developing and refining new, higher-order systems built on top of these foundational components that create new sources of value.
Fig.115: Higher-Order Systems Create New Sources Of Value
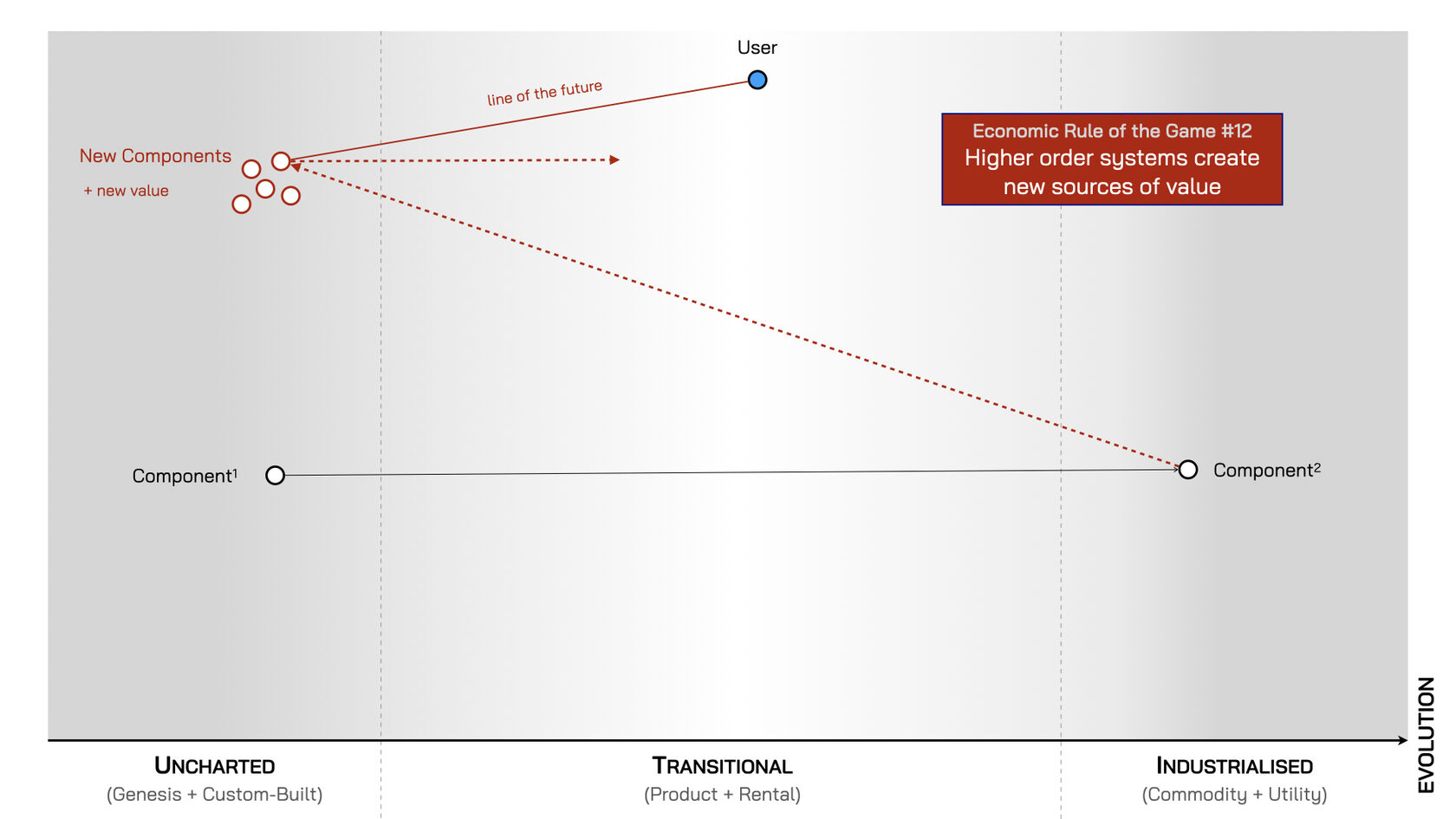
Rule #13. Evolution To Higher-Order Systems Results In Increasing Local Order And Energy Consumption
Running higher-order systems, such as Large Language Models (LLMs), increases the consumption of the underlying components they’re built on, such as computing power and electricity. Yet, before others take the risk of building their higher-order systems on top of these sub-components, they must trust that these platforms are sufficiently reliable and cost-efficient enough to justify the investment. This demand for reliability and efficiency drives the evolution of the underlying components through greater standardisation to reduce deviation in performance and certainty about usage.
However, as these more ordered and standardised underlying components become platforms that others build upon, overall energy consumption rises due to increased adoption and usage — Jevons’ Paradox (see rule #11). Therefore, to maintain margins, providers have to keep innovating, but improvements take place ‘under the hood’ — hidden from end users behind an unchanging standard interface, like a plug for electricity or an API for digital devices — so they continue to deliver what users value most: reliability and efficiency.
Fig.116: Evolution Leads To Increasing Order And Energy Consumption (Jevons’ Paradox)
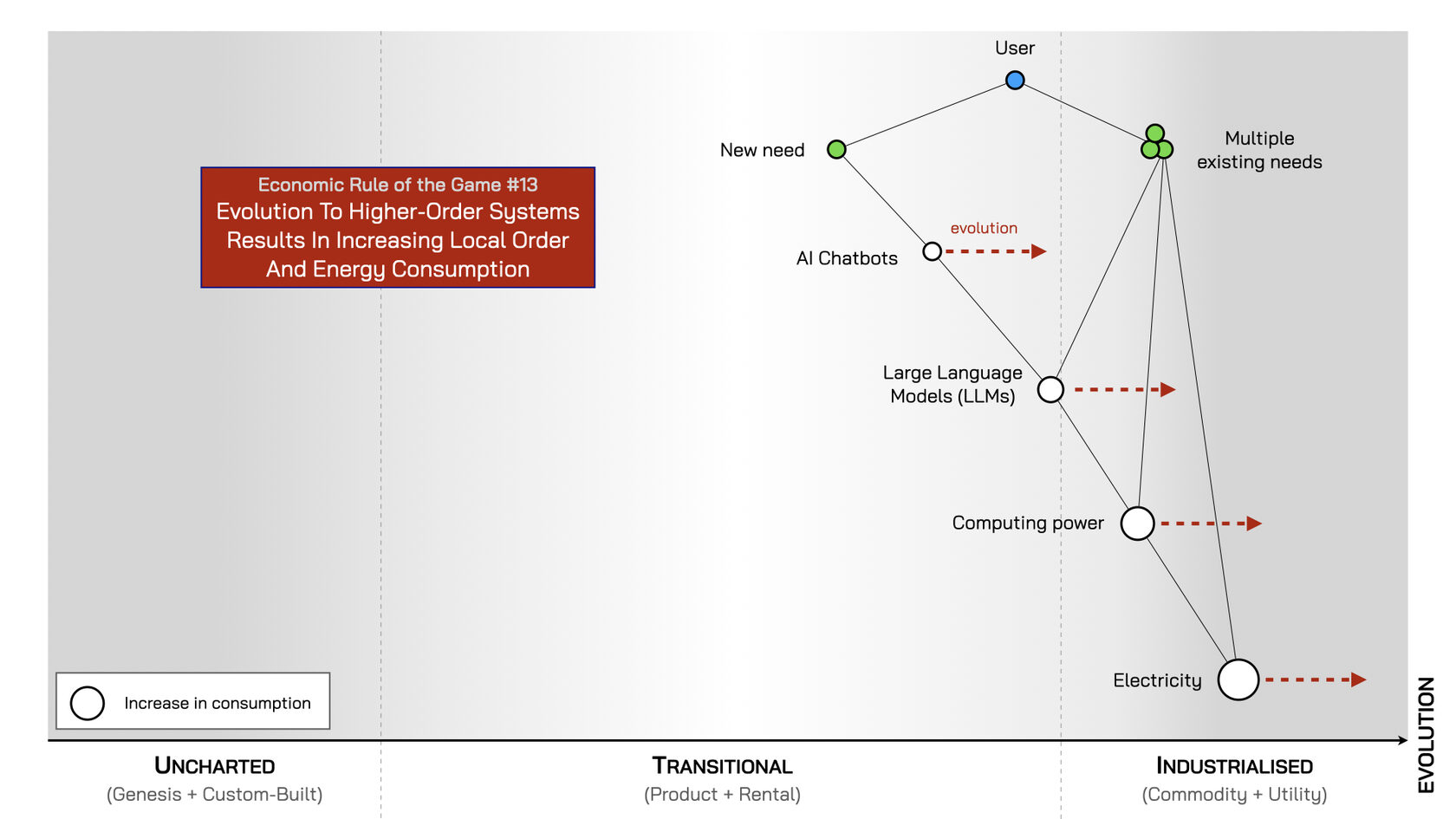
Rule #14. Capital Flows To New Areas Of Value
Financial capital seeks the highest returns on investment, so it tends to flow into components with the greatest potential for future value — and away from components whose value has already peaked.
Once an experimental component evolves from the uncharted space (on the left of the map) into the transitional space (in the middle), where it becomes a product or service in demand, capital flows away from continued experimentation. For example, once VHS video recorders ‘crossed the chasm’ in the 1980s, capital flowed away from further development of alternatives (even technically superior ones like Betamax). VHS had found its market and capital flowed into building the infrastructure and practices needed to make it profitable — from factories building more machines and cassettes to new retail practices like video rental stores (such as Blockbuster).
In the same way, once a product or service matures and moves from the transitional space into the industrialised space (on the right), where it becomes a commodity with lower margins, capital flows away from sustaining the old products and into scaling operations — aiming to deliver utility-like services that generate profits via volume rather than margins. For example, as cloud computing made IT infrastructure cheaper and more reliable, home entertainment was served more conveniently and efficiently by streaming services like Netflix — which ultimately replaced Blockbuster.
Financial capital flows to new areas of value. And, because capital is a finite resource, it invariably flows into tomorrow’s value providers and away from yesterday’s — no matter how successful they were in the past.
Rule #15. Creative Destruction (Joseph Schumpeter)
‘Creative destruction’ — where the rise of the new comes at the expense of the old — was a term coined by the influential Austrian economist, Joseph Schumpeter, in his 1942 book ‘Capitalism, Socialism, and Democracy’.
At the time, the prevailing economic orthodoxy held that markets naturally move towards a state of perfect competition, where many companies sell identical products at ever-decreasing prices. But Schumpeter challenged this, arguing that capitalism “not only never is but never can be stationary”2. He claimed that “the fundamental impulse that sets and keeps the capitalist engine in motion comes from the new consumers’ goods, the new methods of production or transportation, the new markets, the new forms of industrial organisation” — all of which drive economies forward by “incessantly destroying the old one, incessantly creating a new one”. This cycle, which he called Creative Destruction, “is the essential fact about capitalism”3. It is this continual replacement of outdated technologies and practices with the new that, “in the long run expands output and brings down prices”4.
People with new ideas build higher-order systems that create entirely new sources of demand. That demand attracts capital. Combined, they strike — but not merely “at the margins of the profits and the outputs of the existing firms but at their foundations and their very lives”5. The emergence of the new therefore is an existential threat for any who fail to adapt in time.
Fig. 117: The Process Of Creative Destruction
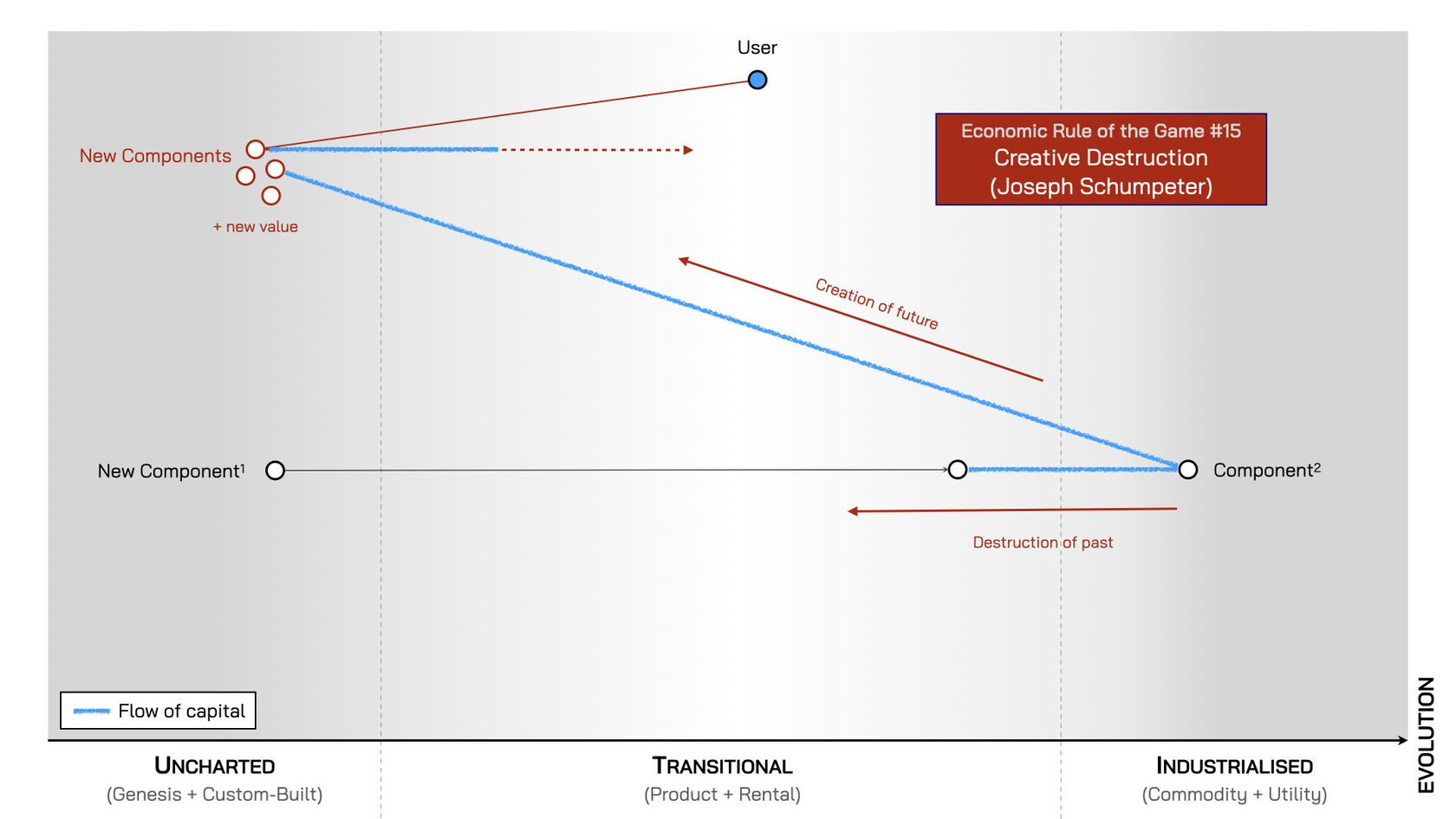
Rule #16. Future Value Is Inversely Proportional To The Certainty We Have Over It
While capital flows to new areas of value, choosing what to invest in always carries risk. There’s an inverse relationship between the future value of a component and the current certainty we have about it.
A component’s potential value is highest when it’s new to the world — shown as being on the extreme left of a map — because all its value lies ahead of it. But this is also when uncertainty is greatest, as the component has yet to find demand and there’s no guarantee it ever will. If it fails to find its market, any investments are lost — making early-stage investing a risky gamble. But when it succeeds, the payoffs can be enormous, as seen with early backers of today’s giant Silicon Valley firms. Picking winners, however, is hard. Success is determined by market forces largely beyond any single investor’s control. As a result, identifying early-stage opportunities is as much an art as a science.
Capital can, of course, be deployed later — once demand is established and the component has evolved further. This reduces risk, because there is now greater clarity about the component and value it’s creating. But that certainty comes at a cost. The price of investment is higher, as valuations reflect the value already being created, and returns are typically smaller. Capital investors therefore face a choice: aim for outsized returns by investing early and embracing the uncertainty; or invest later, when outcomes are more predicable, but accepting that while the risks will be lower, the rewards will be also.
Certainly comes at a price.
Fig. 118: Future Value Is Inversely Proportional To The Certainty We Have Over It
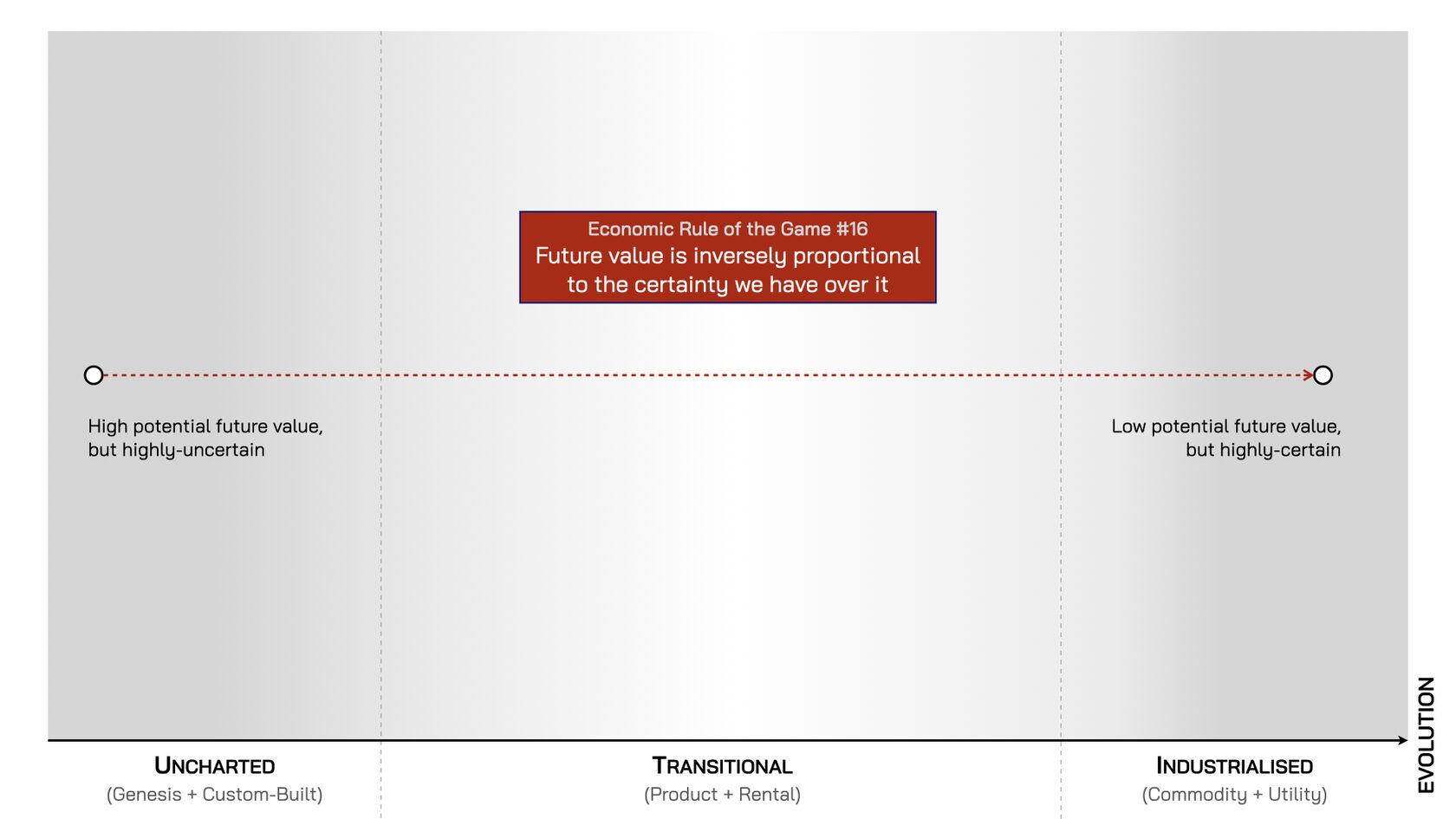
2 Capitalism, Socialism and Democracy. Joseph A Schumpeter. P82
3 Ibid. p83
4 Ibid. P85
5 Ibid p84